An interview with Dr. Adriana San Miguel Delgadillo
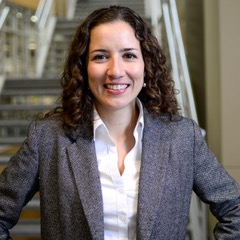
Dr. Adriana San Miguel Delgadillo is an Associate Professor in the Department of Chemical and Biomolecular Engineering at NC State University. I was first introduced to Dr. San Miguel when she gave a seminar describing the beautiful and fascinating mix of microfluidics, machine learning (ML), and biology used in her research. It was the first time I learned of ML techniques being applied to aid fundamental research, and it set off a chain of events that led me to the Institute for Advanced Analytics (IAA).
In our interview, she discussed how her research group uses machine learning to capture and process worm (Caenorhabditis elegans, or C. elegans) images and how these pictures are used to investigate aging, stress, and neurodegeneration.
Ryan: Describe your research for those of us who don’t have a background in biology.
Dr. San Miguel: We try to use engineering tools to study biology, mainly aging and neurodegeneration, in a little model organism called C. elegans, which is a small worm. It has many advantages that make it a really good model to study certain things such as aging and neurodegeneration.
The engineering tools we use involve microfluidic devices which are these tiny chips that allow us to trap our little nematodes that are 1 mm long and 50 microns wide, so very small. Using these microfluidic devices, we can create tiny channels in chips and can control them [the fluid flow in the chip] very well. This makes the handling of these nematodes a lot easier than traditional techniques. We couple our microfluidics for high throughput animal handling and sorting with machine learning to quantify analysis of imaging-based read-outs to create big data sets.
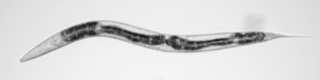
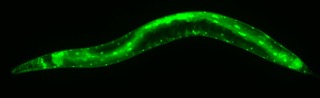
Most of what we do revolves around fluorescence microscopy because one cool thing about these worms is that they are transparent, so we can express fluorescent markers in these worms to label whatever we are interested in, a cell or an entire tissue, and then we can just look through them.
Ryan: How is machine learning used in your research?
Dr. San Miguel: The next thing we want to do is be very quantitative with our images, so can we extract as much information as possible from these images and so that’s where machine learning comes in.
We started with simple ML approaches called support vector machines that are really well-developed. They [support vector machines] are supervised machine learning where you tell the computer, “OK, these are the features I want you to identify in my image.” For example, when we were looking at synapses (connections between neurons or between neurons and muscles), we could label the synapses to train the algorithm and then have the machine learning pipeline identify synapses in new images, which we can then use to characterize them – how many there are, how big they are, things like that.
But there’s a lot of noise in our images because we are looking through an entire animal. So, differentiating the things we want to see, like synapse versus everything else that’s just noise, is not as easy as it sounds, so that’s where we rely on the ML.
We train the computer. We give the computer images that we have hand-annotated and tell it this is what we are looking for. Then the computer comes up with a model to differentiate things that are synapses vs. things that are not.
Those are the initial ones [ML models] that we worked with that were really well established. We have recently transitioned to more advanced models, deep learning, and convolutional neural networks to analyze images as well. More recently, what we have been doing with those is studying neural degeneration induced by aging in these worms. Something that happens with aging in neurons is that they start to show different morphological changes. One of them is the appearance of little bubbles or beads. But a single image from a worm can have hundreds of these beads, and again there is a lot of noise in the image. The computer analyzes the images and tells us there are these many beads, and they are in this specific location that has these patterns.
Then we can go a step further and use additional ML tools to try and fingerprint our worms. That is where we are using the deep learning tools… we can back-calculate the age of a worm from its neuronal morphology. So the initial ML tool tells us, “These are beads, and these are not,” and then the follow-up machine learning algorithm can also tell us, “And it looks like this worm is five days old or seven days old or is about to die,” or something, like that. So, the point of that is really to see how much information this gives us and if this can be a marker for aging.
Ryan: What is the benefit of having these ML tools over how this type of research was done?
Dr. San Miguel: It [ML] really allowed us to look a lot deeper. If you’re going the traditional way, then when you looked at a neuron that was degenerating, what people would do is score it and say healthy, unhealthy, or in between – bin things into three different pots, and that’s it.
When you can get really quantitative, you can get new findings.
To give an example of how ML helps us, we can compare the patterns of different stressors. We compare how aging or something else like a cold shock induces changes in neurons. We identified that the patterns of how these beads are generated are very different. Aging causes beading closer to the center of the worm, and cold shock happens further away. Something that is not obvious at all when you look at the two images. This tells us that it is very likely happening through different biological mechanisms at the cellular level. We think there is cellular transport that happens [due to the different stressors] that could be affected in one but not the other. This is not something that could be seen if you just bin into scores 1, 2, and 3.
Ryan: What research questions do the results from your images help you to answer?
Dr. San Miguel: We are trying to better understand aging, specifically things that go wrong with aging in the nervous system – either aging-induced decline in the nervous system or things associated with neural degenerative diseases that are commonly linked to aging. Some of the things we are trying to understand are
- What are the causes of degeneration with natural aging?
- What drives these changes?
- What can we do to prevent them?
- What are the genes that are important in these pathways?
- How do they [the genes] work together?
We use a working model [the worms] because it gives us a handle to study the genetics of these processes in better detail.
The other thing is whether we can use this model for drug screens. For example, “Can we identify genes that we can drug, other drug targets, or drugs that work to alleviate degeneration induced by aging or other things?” Also, we are looking at protein aggregating in aging and how that intersects with changes in the neural system. We are using a model system because that allows us to look at things a lot quicker than we would with other systems – with the hope that this will give us a handle to look through a lot more genes and drugs faster and have a refined set for studies to be done in higher model organisms. Then we need to collaborate with people who work with mice and higher-order models until we hopefully can impact human health.
Thanks to Dr. San Miguel Delgadillo for taking the time to share her work with me. Her ability to adapt tools from different scientific disciplines is inspiring. Moving forward in my career, I hope to utilize the same approach, selecting analytic techniques appropriate for the current task while keeping the big picture in mind.
Columnist: Ryan Maloney