In the midst of our data ethics courses and the lingering discussions on how we can take the values of ethics into our careers, regardless of industry, I began to wonder what data is like in a sector that encompasses these ethics and, in fact, even focuses on making an impact on society.
I have always been influenced by how data can be used ethically in efforts to make an impact on social issues, and that’s how I came to know DataKind and its employees. I reached out to Rachel Wells, Director of Data Science, Education, to see if she would be willing to talk about using data in the social impact sector and how she uses ethics.
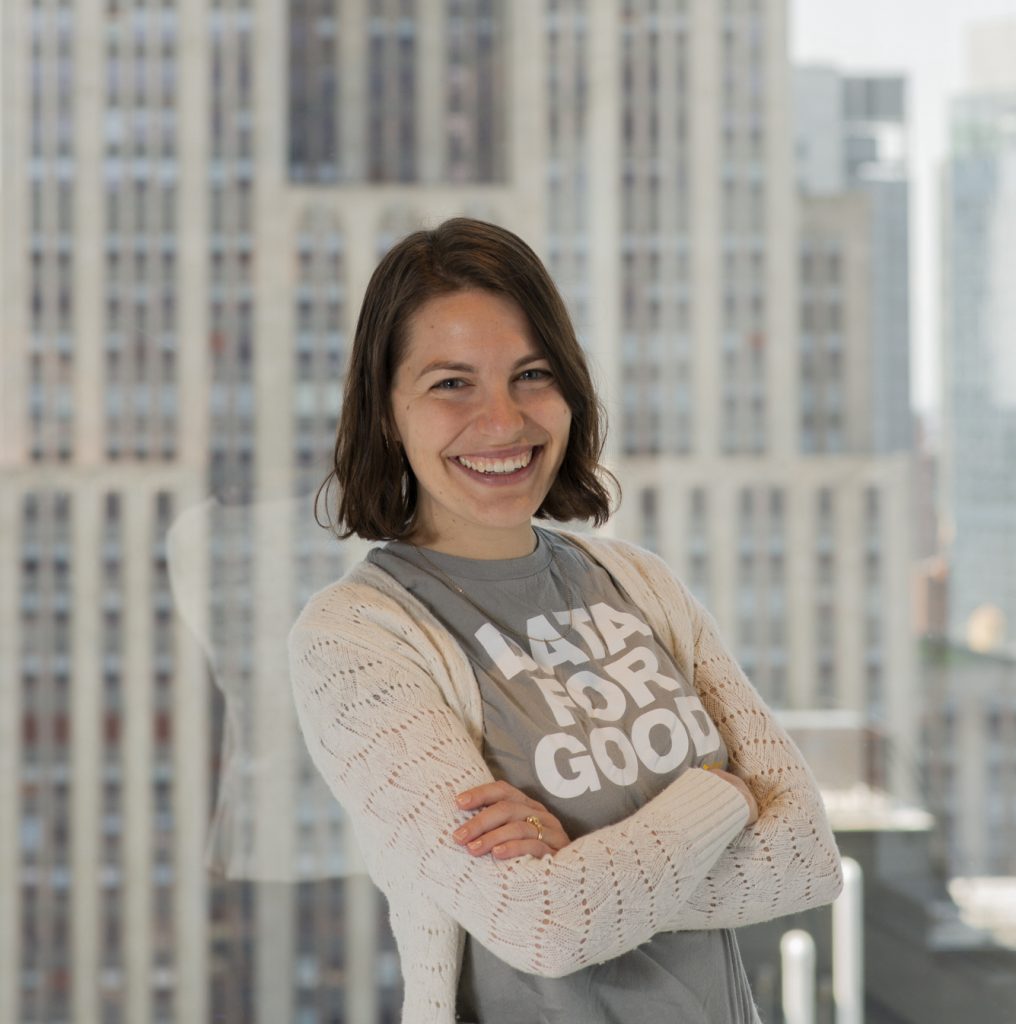
Cameron: How is data used in the social impact sector?
Rachel Wells: It means the same as it does in any other sectors, but instead of having profit as the objective you are working towards, you’re looking for impact. The social impact sector differs from other disciplines in using data because it is much more difficult to measure impact, knowing what is making a positive impact. The weighing of pros and cons of doing something more human and personal, using data science to complement that, matching the situation’s needs to the right data science approach, and really thinking carefully about the impact.
Measuring the impact has been a big challenge in the social sector. Data is an amazing tool to help move forward in that way, but it’s incomplete, and there’s a lot of complementary work needed too. It’s a really complicated space to optimize the impact rather than profits.
Cameron: You covered a lot about the difficulties behind measuring the success of social impact. When you attempt to measure it, is this something you personally do? Or is this a company-set standard?
Rachel: It varies; there isn’t one correct approach. Every project is so different, and the products have different needs. In many cases, we are using data to measure a program’s social impact. An everyday use case for data in the social sector is to take an existing program and measure its impact. However, if we are looking at a data science intervention, like our student success tool, it is not an impact evaluation; instead, it is a data science tool that’s meant to be the intervention. I will note that historically, data has been used mostly in the social sector for evaluation purposes, but now we are seeing where data is the intervention.
Our student success tool is where we are building a tool that helps advise or identify students who might be at risk of not graduating, looking at their behavior or school data, and trying to identify those key factors of potential risk and seeing who might need more support from the advisor. The tool is meant for the advisor to use as a complementary tool to know what support is helpful and who may need it.
We have a fabulous research agenda for this particular tool that we are working through alongside our intervention and tool development. We are writing tests and evaluations with the schools that are using the tool and are looking at some possible randomization of enabling the advisors with the tool at a couple of schools to see if the graduation rates increase for advisors who use the tool versus those who don’t. We are also looking at some really thoughtful study designs to ensure we have a strong methodology for standard monitoring evaluation methods for social programs.
For this program, we are focused on measuring the impact of the tool comprehensively, which is exciting to have alongside our tool development. However, there are many times that we don’t have the budget to do that, so we have to do something light lift, where we do a simple interview with the partner at the end to get an idea of its impact. We ensure that we consider bias and equity in all of our evaluation measures.
Cameron: I was hoping to hear about some projects that were going on, so that was a great insight into the current student success tool. In the end, you mentioned thinking about biases; how do you handle the biases you may encounter during modeling?
Rachel: There is always some bias in data and society, and using the student success tool again, I can share examples of them. In one school, they might see that women generally have a higher graduation rate than men. A tool may predict that men are at higher risk of not graduating than women. That would accurately represent that sample, but does that mean it’s appropriate to use gender as a variable?
We test our models for each of the demographic variables we have, look at the outcomes they provide, and make sure they enable support for a variety of groups across all the features and that rates are not massively different from the data we see. If we see more women graduating, then we should expect to see more men in our classified “at risk” group, but we don’t want to see a ratio that is not reflective of that, especially for the potential at-risk women who need the support.
One thing that was the norm in data science focused on equity and bias, maybe about five or six years ago, was not using demographic variables. It was this sense of being color-blind, like with the anti-racism language, and this isn’t the answer because then you don’t know about the biases that are persisting. DataKind has developed an approach where we let the models be “color-blind” so that it can’t classify people based on demographics, but we always make sure we have data on demographics, and in the evaluation stage, we check for equity.
Cameron: Many of these comments you’re bringing up about equity and biases, and understanding those impacts through different processes of your work is insightful, especially as someone who wants to ensure they carry these values with them as a data professional. I was curious how you might think data professionals not in the social impact sector can still implement these ethical values and ideas in their products or work.
Rachel: We are seeing more and more in our society that people working in data science and AI want to think about ethics and bias across sectors. That is so encouraging, and there are so many resources out there on how to do that best, although some of them are simpler and more box-checking rather than as in-depth as we consider for the social impact work. It’s a different space than the social impact space, and that’s because a lot of it comes down to the end result, and a majority of other industries’ end result is profit. Overall, there are so many resources and approaches to ethics and biases, so just utilizing these to understand what works best can help.
End
Rachel shared lots of valuable insights on utilizing data for social impact and navigating potential biases. Moving forward, I’m eager to explore resources on bias mitigation and investigate the industries that interest me. As I continue my journey to enter the data professional world, I’m committed to implementing ethical values in my work and inspiring others to consider ethics, biases, and social impact.
Columnist: Cameron Houston