If there is anything my time at the Institute for Advanced Analytics (IAA) has taught me, it is to trust the process. Throughout the past 4 months, I have expanded my knowledge of data science under the tutelage of amazing faculty and staff. They have pushed me to process information in innovative ways to gain understanding of potential underlying meanings in data. Within the IAA’s environment, I have also challenged myself to become a better communicator in terms of presenting to people and conveying thoughts in group settings. These are skills that I have drastically expanded on here at the IAA, but the introduction of these skills was fostered in my undergraduate career in biostatistics.
My background in biostatistics fostered a personal area of interest for me in navigating the world of public health with an analytic focus. I was given an introduction to what a career in public health could look like, and that was very appealing to me. I wanted to focus more intently on an application-focused graduate degree in order to hone my technical skills, and this is what led me to the IAA.
In my career after graduating from the IAA, I hope to spread health information that is backed by mathematical principles. In order to explore some themes related to this interest, I asked Dr. Michael Hudgens, professor and associate chair of the UNC-Chapel Hill department of biostatistics, to share some of his insights. He has spent time focusing on AIDS research and serves as the director of the Biostatistics Core of the UNC Center for AIDS Research (CFAR). His background in education is from the University of Florida for his bachelor’s and master’s in mathematics, and Emory University for his PhD in biostatistics.
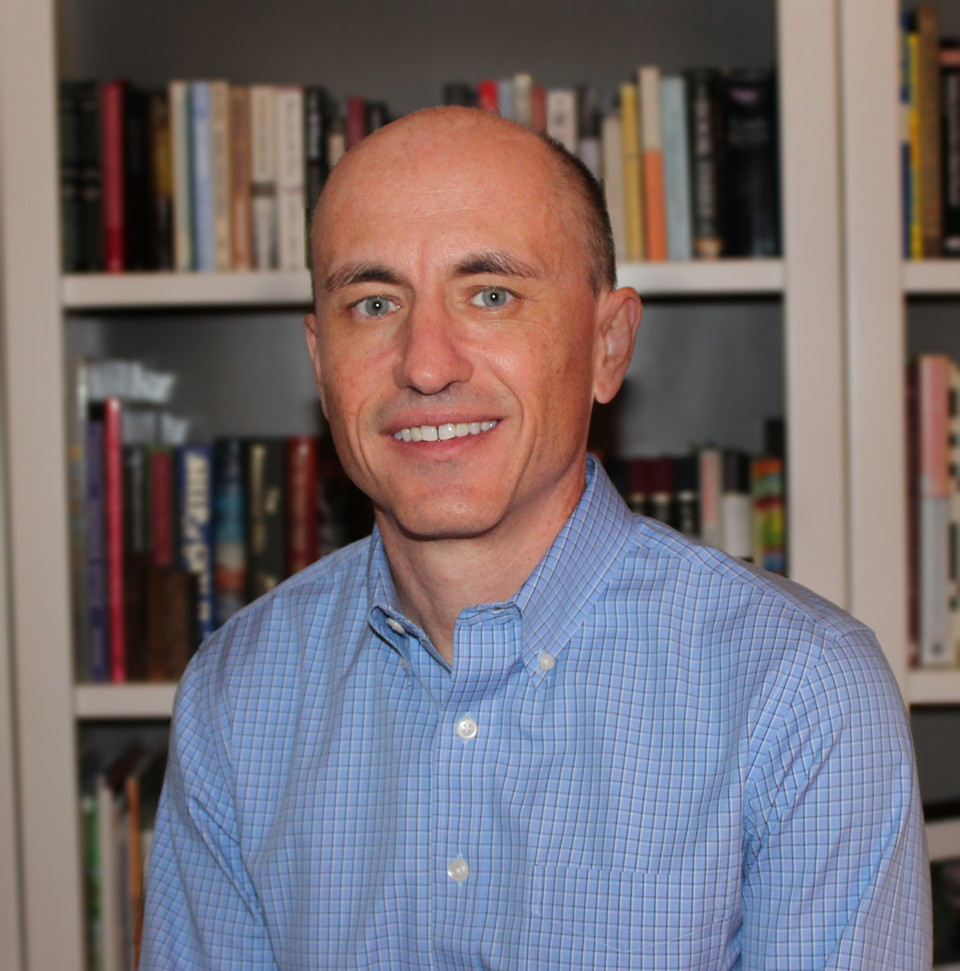
Lexi: What initially spurred your interest in public health, and in particular, biostatistics?
Dr. Hudgens: I was a math major as an undergraduate and master’s student at the University of Florida, and I was on the path towards getting a PhD in math. As the courses became more theoretical, I started to lose interest in them and started looking for some future path that was more tied to the real world and a little less abstract.
During the master’s program, I took a course on biomathematics, focused on application of mathematical modeling to different applied problems in biology and ecology. It was very interesting and eye-opening to see math used in this way. I looked for grad programs in biomathematics and found that there were also programs based in biostatistics, which I had not heard of before. I was fortunate enough to get into the biostatistics program at Emory, and that’s really the genesis of how I got involved in this field and have enjoyed it ever since.
Lexi: In what ways have you found statistics and analytics to be useful when discussing AIDS in your role with Center for Aids Research (CFAR)?
Dr. Hudgens: I’ll answer that question maybe not as directly as you have phrased it. Why am I involved in HIV research is probably a question I can answer more directly, and that dates back to when I started at Emory. Some of the faculty members there had a training grant on biostatistics in HIV, and I was placed on that training grant. It seemed important, and the sort of problems that I thought were exciting to work on. It was motivation to get up out of bed in the morning and spend time and energy towards dedicating to this kind of research. Once you get training in a particular area, it can open certain doors, so when I was looking at postdocs and faculty positions after graduating from Emory, I was given an opportunity to join the Fred Hutch Cancer Research Center in Seattle, where they were conducting HIV vaccine trials. To me, this seemed like exciting research to be a part of. I joined that team and worked there for four years.
When coming to UNC, having all of this experience, it was very natural for me to work with the Center for AIDS Research here at UNC. I have been a part of CFAR since I joined in 2004, and I have been directing the Biostatistics Core since 2010. From a statistician’s perspective, being part of the Biostatistics Core is really fun, interesting, and challenging. The Core of the CFAR serves investigators from UNC, FHI-360, and RTI working in HIV, and anyone who needs analytical support can come to the Core. From the Core’s perspective, we’re faced with all kinds of different statistical problems. Maybe investigators will come to us with small datasets, maybe there are pre-clinical studies of HIV candidates where the n is very small, but this does not mean the p is small. We also work closely with epidemiologists and clinicians that have huge cohort studies and everything else in between. This requires us collectively to have a large skillset among the members of the Core. Folks who work on the CFAR Bios Core have opportunities to work on projects related to other infectious diseases also. Recently the Core has been involved in projects related to Covid, but prior to Covid, we have gotten involved on projects with flu, HPV, and Zika along with other viruses.
Lexi: I saw two of your interests are Survival Analysis and Causal Inference. How do these two interests pertain to data science in public health?
Dr. Hudgens: Survival analysis is a very mature older area of biostatistics. People have been working on this problem for decades; the famous Kaplan Meier estimator goes back to the middle of the last century, and the Cox model is almost as old as I am. Time to event analysis shows up throughout public health studies and in AIDS-related research also. For example, in a preventative HIV, the endpoint might be time until HIV infection. In studies of individuals living with HIV, the endpoint may be time until AIDS or some other clinical endpoint. We sometimes use viral load as a surrogate, so time until viral suppression or time until viral rebound can also be used. Time to event is often the primary outcome in these studies. It is common in randomized control trials and also observational studies as well. This kind of problem is seen over and over again. Thus survival analysis continues to be important, and any practicing biostatisticians need to have a really good handle on that topic.
Regarding causal inference, we’re told in our training as statisticians that association doesn’t imply causation, and that’s not a bad rule of thumb, but under what circumstances can we infer causality from association? I’ve been interested in this problem for a long time, and after I’d been at UNC for a few years, Steve Cole joined the faculty. He had been at Hopkins, and he was also very much interested in causal. We have been working together and growing the causal group here at the UNC Gillings School of Global Public Health since that time. We have a causal inference seminar series that’s had over 100 speakers now come and give talks on causal inference, with presenters locally from UNC in the triangle area and also from beyond.
We have also developed a causal course and lab, and we have been fortunate enough to receive methodological grants where specifically Steve and I and others in our group are interested in the intersection between causal inference and infectious diseases. From this, we have tried to develop and employ causal inference methods with application to infectious disease studies. For example, some of us work on causal inference methods to analyze the causal effects of vaccines. In particular, we are interested in so-called herd immunity or spillover effects, meaning whether or not one individual gets vaccinated can affect the outcome of another individual. We’ve been working on that problem for quite a while.
Another problem specifically we are interested in is the generalizability problem. It’s often the case that we have data from some kind of convenience sample, so it’s not a random sample from a target population. How does one estimate the effects of a treatment or exposure from a convenience sample? For example, suppose you ran an RCT (randomized clinical trial) such that you would have the benefit of randomization. Causal inference is easy in an RCT. However, RCT’s enroll a kind of a convenience sample, meaning whoever is willing to show up and partake in the RCT is included, so it’s not truly a random sample from a formal target population. So, you’ve got this RCT, where there is so-called internal validity that you can draw valid inference about the effect of the treatment from the trial because of randomization, but that doesn’t necessarily mean that you’re getting the right answer about what the truth will be in the target population because of this population for sampling bias, or selection bias. That’s an area folks have been working on here for years: How do you generalize those results, scale them up to a target population of interest?
Lexi: What links do you believe there are between biostatistics and analytics/data science as a whole?
Dr. Hudgens: There’s been a lot of discussion about “What is data science?” People spend a lot of time thinking about that. I’ve heard people say that biostatistics is data science, and I think that is accurate. You can say that it is a field within data science. Data science seems quite broad, so I think of biostatistics as a type of data science focused on biomedical and public health research. But certainly, a lot of the tools you use in biostatistics are the same as what most would consider the tools of data science.
In the larger realm of data science, we think about tools that are beyond what we would consider for biostatistics. In biostatistics, there is a little more emphasis on statistical methodology, theory, inference, and maybe a little bit less emphasis on the computing side of things. Not to say that we don’t take that seriously as well, but (historically anyway) there is more focus in biostatistics on the analytics side, and less on the data wrangling side. Certainly, folks in biostatistics acknowledge this is an important part of the process as well. For instance, we now have multiple data science courses within our department recognizing the importance of this for our students.
Overall, there is a direct link between the principles that are used in the field of biostatistics and those that are used in analytics.
Based on what Dr. Hudgens has said, biostatistics is just a more niche field that is based around the ideas that are used in analytics. In this way, my education in analytics allows me a broad scope to jump back into public health after graduation from the IAA if that is the career journey I should be interested in. This program was also an important choice for me if there are other compelling fields I wish to explore after continuing my education. The IAA has given me the chance to spread my wings.
Columnist: Lexi Bell